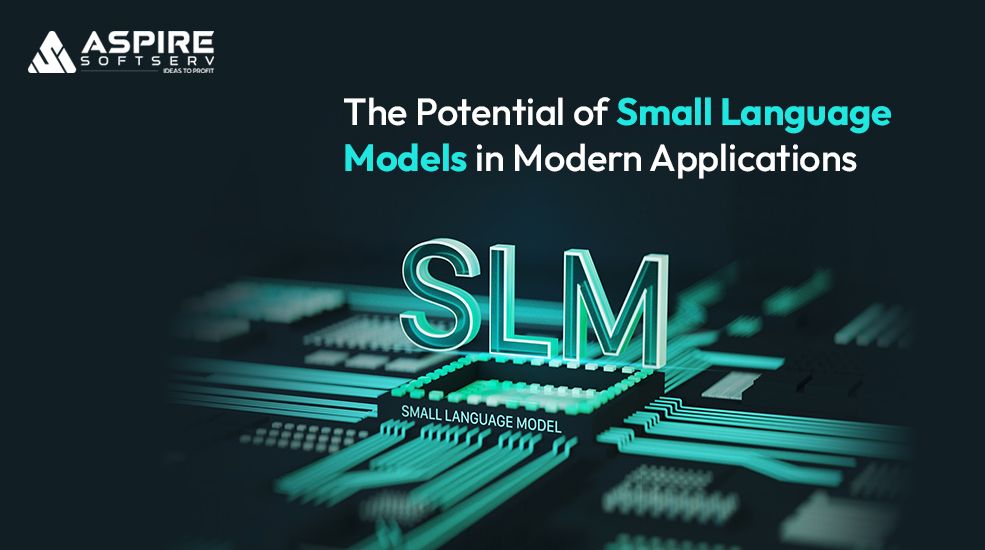
With the evolution of artificial intelligence, powerful tools for natural language processing (NLP) such as GPT-3 and GPT-4 have been introduced. Since then, these large language models (LLMs) have gained a lot of attention because of their powerful ability to generate human-like text, comprehend the context, and execute a range of different tasks. But with an increasing need for low-accessibility, fast, and green AI solutions, small language models (SLMs) are becoming more prevalent.
Notwithstanding their relatively small size and lower demands for computation, these models pack a lot of potential for contemporary applications across verticals.
Small Language Models: What Are They?
There are many of them, and they are some small counterparts of the large natural language processing (NLP) models that work on fewer parameters and computational resources to do one task. Before you think, sure, GPT-3 and the other large models are cool, but they have billions of params under the hood, most small models aim to be far more lightweight, optimised and optimised again. This enables them to complete comparable operations at a fraction of the energy usage, higher inference speed, and lower expense. While small language models may not always reach the wide range of skills that very large models have, they do a very good job for narrower applications and usage contexts and can be quite effective if they are trained on specific examples. Utilisation of Small Language Models in Solutions.
1. Edge Computing and Real-Time Processing
Small language models work best for implementing them where edge computing is performed, where data is processed by the device rather than relying on the whole cloud server. These may be applications such as voice assistants on mobile phones, AI-based intelligent devices, embedded systems, etc. Small models can even do NLP tasks—like speech recognition, sentiment analysis, or text generation—right on the devices, which means that they don’t require a consistent internet connection.
For example, virtual assistants such as Siri and Alexa use cloud-based models for more complex queries. But smaller language models might allow devices to carry out simpler tasks at the edge, improving response time and creating a more seamless user experience. The advantage is that real-time processing would be quicker, more reliable and more private, because the user’s data wouldn’t have to be sent for processing to the cloud.
How This Helps:
More responsiveness: Devices can process text or voice commands immediately without responding to a cloud query.
Faster response times: Devices can process text or voice commands immediately rather than querying the cloud and waiting for a response.
Independence from an Internet connection: Small models can provide offline apps, which increases their reliability.
Enhanced security: Performing analytics on-site eliminates the transmission of sensitive data to cloud-based servers. Example Applications: Voice Assistants (Siri, Google Assistant): Solution-oriented for simple commands, executed locally, narrator not hosted on a server.
Example Applications:
Voice Assistants (like Siri, Google Assistant) have the capability of executing simple commands locally, and without you having to connect to the internet.
AI driven cameras and Internet-connected devices analyse the text-based inputs in the real-time.
Some tools that perform transcription in mobile apps can work without cloud-related processing.
2. Cost-Effective AI Solutions
Scaling up large architectures, like GPT-3 or GPT-4, requires a lot of computing power and thus expensive operational and energy costs. The smaller dosser options are vastly less expensive to train, deploy and maintain. These solutions are very cost-effective, because of which they are quite attractive to startups, smaller businesses and individual developers who are willing to add AI capabilities to their products at a low-cost. However, small language models can anachronistically power applications with large models (chatbots, automated customer support systems and language translation tools apart) being able to perform admirably without expensive on-demand cloud infrastructure or bespoke hardware. With access to AI becomes democratised, companies big and small can pick up and advance with NLP technologies.
How This Helps:
Lower development & deployment cost: Organizations can now easily have AI integration under less investment and don't have to spend on heavy infrastructure.
Better scalability: The saturation of system resources is not a problem when deploying small models on separate devices.
Easy maintenance: Since it has fewer parameters; it trains and updates more quickly.
Example Applications:
Small business customer support chatbots offering automated responses without using a large cloud-based model.
E-commerce businesses making use of AI-driven recommendations — but not the overhead of LLMs.
AI language and tutoring solutions for education that are not expensive to run on servers.
3. Faster and More Efficient Processing
Speed is essential for real-time applications. Small language models are able to be processed more quickly than their larger counterparts since they have fewer parameters to evaluate. This enables them to react to what the user writes in real-time and is critical for applications such as conversational agents, chatbots, or any Artificial Intelligence solution that interacts directly with users.
As an illustrative example, think of a customer support chatbot. The bot understands user queries quickly and generates a response right on the device without the delay of having to call the cloud like in the case of a small language model. Likewise, AI-driven autocomplete or autocorrection in chat applications relies on the nimbleness that small language models offer.
How This Helps:
Improved user experience: Instant answer means a seamless experience.
Lower Latency: This is critical for apps such as virtual assistants, real-time analytics, etc.
Major new features include Mobile app improvements: Text prediction, translation and summarization, faster than existing machines.
Example Applications:
Chatbot based on conversational AI: for quick responses in messaging apps
AI-based email helpers: it can suggest responses, in real time.
Search engines: smarter producing quicker and better-relevant results.
4. Improved Data Privacy
Data Privacy is one of the growing concerns with large-scale language models. There are security risks with user data being sent to the cloud for processing and questions over how that data will be stored and used. In contrast, smaller language models can be directly embedded on devices, meaning user data remains on the device and is not sent to external servers.
For example, this is critical in sectors such as healthcare, finance, and legal services, where sensitive data must be dealt with extreme caution. Small language models can maintain the privacy needed by these industries while delivering enough NLP capabilities.
How This Helps:
Turns off external data sharing: You’re not texting queries to a remote server.
Enhanced regulatory compliance: Like privacy laws such as GDPR and CCPA, data stays on-device.
Safer applications of AI in healthcare and finance: Sensitive data is preserved.
Example Applications:
Private, cloudless virtual assistants that do not share user speech data.
Healthcare AIs that process patient data on premises, on hospital systems.
AI-based personal finance mobile apps analysing users spending without letting their data get exposed on the web.
5. Sustainability and Environmental Impact
One of the stinging questions regarding the environmental cost of the training process of large models has been a hot topic. Training a large-scale language model consumes a lot of energy, which causes a large carbon footprint. Lightweight language models are a much more sustainable choice, demanding far less energy during training and deployment. This means they do not lose out on the benefits of AI, while actively reducing the negative impacts that they have on the environment. Small language models are a clear advantage in applications where extreme energy efficiency is a consideration, such as AI-enabled devices that run on limited battery power or where the supply of electricity is intermittent, or simply unavailable. With lower amounts of energy needed for each transaction, they can provide a more environmentally friendly alternative in line with global attempts to reduce carbon emissions.
How This Helps:
Less energy consumption: Small models consume far less energy.
Lowered Carbon footprint: Sustainable AI Development.
Improved accessibility of AI: Enables deploying AI in energy-limited (edge) environments.
Accessibility of better AI: Enables AI deployment in energy-constrained environments.
Example Applications:
Battery farmed powered IoT devices with low-power AI assistants.
Low-compute AI research projects for sustainability.
Government and NGO machine learning initiatives focused on sustainability.
6. Personalization and Domain-Specific Tasks
Large language models capture a wide spectrum of language patterns, but small language models can be customised for specific industries or tasks, making them worthy performers in one-off applications. Models that have been fine-tuned for a domain (e.g., healthcare, legal, finance, education, etc.) will be more accurate and relevant than the broader-based models.
A small model that can be fine-tuned for legal jargon could help analyse the contract or write legal documents. In a similar fashion, focused small models on medical vocabulary can support healthcare providers by processing patient data, summarising clinical notes, or offering decision support. You are fine-tuned for a specific domain, as such, the output generated by the model is more relevant which allows for better and faster results.
How This Helps:
Improved accuracy in fields of specialty: Can be tailored for legal, medical, or financial apps.
Enhanced relevance: Raises more industry-centric contextual responses.
More effective AI solutions for anything else: Specific models beat generic models.
Example Applications:
AI legal models that review contracts and produce legal documents.
Medical artificial intelligent assistants that offer treatment recommendations based on medical literature.
HR software that uses AI to screen resumes against industry specific positions.
7. Enabling NLP in Low-Resource Languages
One of the drawbacks to large language models is their dependence on a large amount of high quality training data. Small language models, on the other hand, can be trained on less data and are thus better suited for low resource languages, languages of which abundant data might not be available. International news and information sharing can thrive, and regions and languages that are not seen as economically or academically viable can now be served with nature language processing (NLP) technology and potentially get a much-needed leg up.
Smaller language models can be trained on languages that have little digital presence like much of Africa and the Middle East, making translation, transcription and sentiment analysis possible where they have not been before. This also contributes to closing the digital gap and enabling speakers of underrepresented languages to reap the benefits of AI-driven tools.
How This Helps:
Underserved languages supported: Backs communities with few digital options.
Availability: Extend AI usage to underdeveloped infrastructure.
Maintain the Multilingualism: It provides the digital presence of smaller languages.
Example Applications:
Translations using AI for regional, Indigenous or African languages
Endangered languages text-to-speech machine learning models).
Prediction for low-resource languages in smartphone keyboards.
Real-World Use Cases for Small Language Models
Smartphones and wearables: Google Assistant and Apple Siri are in fact working on small language models allowing real-time processing on the device.
Customer Support with Chatbots: Smaller models are used to power service-oriented chatbots across sectors such as e-commerce, banking, and telecommunications, allowing these systems to respond to customer questions quickly and accurately.
The healthcare domain: Health care domain-specific NLP models can help in the analyse patient records support systems for diagnosis and real-time information delivery to health care professionals.
Teaching aids: Small language models can be used in personalised learning applications to help students with grammar fixes, language learning and homework.
IoT and Smart Devices: These small models are used in smart speakers, smart thermostats, home assistants, etc., to perform tasks like speech recognition and natural language commands.
Conclusion
With small language models, we have a viable solution for many modern applications, and they can operate on a smaller scale. They can run on edge devices, process in reasonable quickness, be fine tuned and thus they are great for your use case concerning real time, domain and privacy sensitive applications.
As technology evolves, small language models will likely help democratise AI and expand its use across industries, languages, and use cases.
Bring AI to the Edge with Small Models